05 Nov 2020
kRadar++: Coarse-to-fine FMCW Scanning Radar Localisation
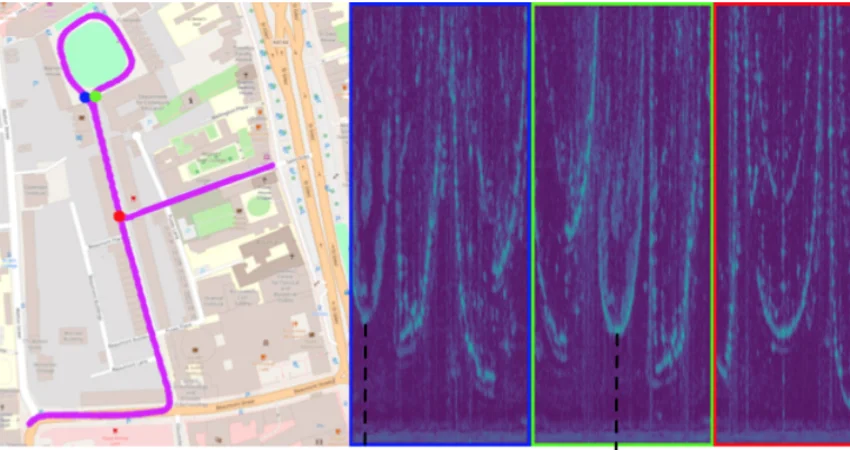
Abstract – This paper presents a novel two-stage system which integrates topological localisation candidates from a radar-only place recognition system with precise pose estimation using spectral landmark-based techniques. We prove that the—recently available—seminal radar place recognition (RPR) and scan matching sub-systems are complementary in a style reminiscent of the mapping and localisation systems underpinning visual teach-and-repeat (VTR) systems which have been exhibited robustly in the last decade. Offline experiments are conducted on the most extensive radar-focused urban autonomy dataset available to the community with performance comparing favourably with and even rivalling alternative state-of-the-art radar localisation systems. Specifically, we show the long-term durability of the approach and of the sensing technology itself to autonomous navigation. We suggest a range of sensible methods of tuning the system, all of which are suitable for online operation. For both tuning regimes, we achieve, over the course of a month of localisation trials against a single static map, high recalls at high precision, and much reduced variance in erroneous metric pose estimation. As such, this work is a necessary first step towards a radar teach-and-repeat (RTR) system and the enablement of autonomy across extreme changes in appearance or inclement conditions.
Further Info – Please take a look at our paper: